Speaker
|
Biography
|
Abstract |
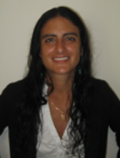
Sofia Villar |
Sofia is an MRC Investigator in the clinical trials methodology group, part of the Design and Analysis of Randomised Trials (DART) theme at the MRC Biostatistics Unit (BSU), University of Cambridge.
Sofia has a Ph. D. in Business Administration and Quantitative Methods at Universidad Carlos III de Madrid in July 2012, with a focus on Stochastic Dynamic Optimization. In 2013, she joined the BSU in Cambridge as part of a project on the design of multi-arm multi-stage clinical trials. In 2014, she was awarded the first ever Biometrika post-doctoral fellowship. Since the end of her fellowship she has been leading a team of statisticians at the BSU and at Papworth Hospital trials Unit. Sofia is currently working on a research programme that aims to improve clinical trial design through the development of innovative methods that lie in the intersection between optimisation, machine learning and statistics. A main focus of her research is to develop statistical methods for patient-centric adaptive clinical trials.
|
Response-adaptive randomization in clinical trials: a whistle-stop tour of myths and barriers.
Response-adaptive randomization (RAR) is part of a wider class of data-dependent sampling algorithms, for which clinical trials have typically been used as a motivating application. In that context, patient allocation to treatments is defined using the accrued data on responses to alter randomization probabilities, in order to achieve different experimental goals. RAR has received abundant theoretical attention from the biostatistical literature since first proposed in the 1930's and has been the subject of numerous debates. Recently it has received renewed consideration from the applied community due to some high profile practical examples and its widespread use in machine learning. In this talk, I will present a broad roadmap of the specialised literature, mentioning some of the most recent methodological work and discussing potential remaining barriers and practical issues to consider when debating the use of RAR in clinical trials.
The talk will be based on the recent review article (accepted for publication in Statistical Science) and available at https://arxiv.org/pdf/2005.00564.pdf
|
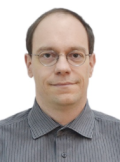
Frank Bretz |
Frank Bretz is a Distinguished Quantitative Research Scientist at Novartis. He has supported the methodological development in various areas of drug development, including dose finding, estimands, multiple testing, and adaptive designs. He was a member of the ICH E9(R1) Expert Working Group on 'Estimands and sensitivity analysis in clinical trials' and currently serves on the ICH E20 Expert Working Group on 'Adaptive clinical trials'. |
Our personal views on adaptive designs. |
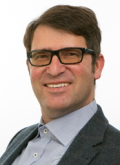
Uli Burger |
Uli Burger has more than 30 years of industry experience at Roche in many therapeutic areas and all phases of drug development, and is currently global head of data and statistical sciences for Neuroscience there.
He has been involved in many statistical communities in organizing seminars and trainings including ROeS (Swiss Austrian region of the in international biometric society), EFSPI (European federation of statisticians in pharmaceutical industry), BBS (Basel Biometric Society) and DIA. Uli has been president of ROeS and EFSPI in the past, is the current president of the BBS and is also a member of the ICH E20 writing team.
|
Our personal views on adaptive designs. |
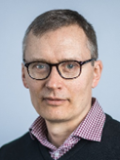
Carl-Fredrik Burman
|
Carl-Fredrik “Caffe” Burman has been working 27 years in the pharmaceutical industry. He is Senior Statistical Science Director in the methodology group, Statistical Innovation, within Data Science & AI at AstraZeneca R&D Gothenburg, Sweden. Most of his work is spent at the Trial Design & Modelling Centre, providing design support to project teams. Dr Burman is associated professor in Biostatistics at Chalmers University and associated editor for JBS. His research interests are mainly within design of clinical trials, decision analysis and multiple inference.
|
Adaptive Designs with Multiple Objectives.
Regulators typically require strict control of the overall Type 1 Error (alpha) in confirmatory clinical trials. In adaptive designs, we often have to control alpha both for 1) repeated analyses (when we may win at an interim) and 2) for multiple objectives.
To control alpha for repeated analyses, group-sequential methodology can be carried over to more general adaptive designs. To control alpha when several null hypotheses are tested, we may use e.g. Bonferroni, Hierarchical, Holm or Dunnett procedures. The mathematical structure for the Type 1 Error is similar for these two potential sources of alpha inflation. Yet, there are important differences.
Even for statisticians familiar with group-sequential alpha spending as well as testing of multiple hypotheses, the combined problem is often challenging. We will therefore discuss how one may think when constructing testing procedures that control alpha over both multiple analyses and hypotheses. |
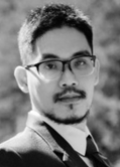
Anh Nguyen |
Anh Nguyen is a Principal Statistical Scientist at Roche. He joined Roche in 2016 after attaining his PhD, and during his time at Roche he has made many significant contributions to disease area strategy, as well as in statistical, clinical and regulatory interactions across a number of molecules, including Herceptin, Tecentriq, Tiragolumab and recently in his role as Project Lead Statistician for gynecological cancers. Anh has also been involved in a number of pan-project activities within Data Science, including biomarker analyses for Triple-negative breast cancer data, overseeing statistical activities in the anti-drug antibodies and neutralizing antibodies working group as well as devising pragmatic adaptive designs. |
Adaptive Enrichment Design to Address Emerging Uncertainty Regarding Optimal Target Population.
One of the challenges in the design of confirmatory trials is to deal with uncertainties regarding the optimal target population for a novel drug. Adaptive enrichment designs (AED) which allow for a data-driven selection of one or more prespecified biomarker subpopulations at an interim analysis have been proposed in this setting but practical case studies of AEDs are still relatively rare. We present the design of an AED with a binary endpoint in the highly dynamic setting of cancer immunotherapy. The trial was initiated as a conventional trial in early triple-negative breast cancer but amended to an AED based on emerging data external to the trial suggesting that PD-L1 status could be a predictive biomarker. Operating characteristics are discussed including the concept of a minimal detectable difference, that is, the smallest observed treatment effect that would lead to a statistically significant result in at least one of the target populations at the interim or the final analysis, respectively, in the setting of AED. |
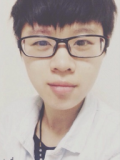
Haiyan Zheng |
Dr Haiyan Zheng is a CRUK Research Fellow in Statistical Methodology based in the MRC Biostatistics Unit. Her research is mainly focused on early phase clinical trials and adaptive methods for precision medicine. Haiyan currently leads a three-year research programme to develop statistical methods that (1) simultaneously evaluate treatment effects in multiple subgroups, and (2) allow for mid-course adaptations in master protocol trials.
|
Design and analysis of basket trials to enable added efficiency.
Basket trials are increasingly used for the simultaneous evaluation of a new treatment in various patient subgroups. Eligible patients would share a commonality (e.g., a genetic aberration or clinical symptom), on which the treatment may potentially improve outcomes. A few sophisticated analysis models, which feature borrowing of information between subgroups, have been proposed for enhanced estimation of the treatment effects. Yet development of methods to choose an appropriate sample size appears to fall behind. A widely implemented approach is to sum up the sample sizes, calculated as if the subtrials are to be carried out as separate studies.
In this talk, I will introduce a Bayesian model that characterise the complex data structure of basket trials, where patients are randomly assigned to the experimental treatment or a control within each subtrial. More specifically, this approach reflects the concern that the treatment effect in some subsets of the trial may be more commensurate between themselves than with others. Closed-form sample size formulae are further derived to enable borrowing of information between commensurate subtrials. Our approach ensures that each subtrial has a specified chance of correctly deciding whether the new treatment is superior to or not better than the control by some clinically relevant difference. Given fixed levels of pairwise (in)commensurability, the subtrial sample sizes are solved simultaneously. This solution resembles the frequentist formulation of the problem in yielding comparable sample sizes for circumstances of no borrowing. When borrowing is permitted, a considerably smaller sample size is required. I will illustrate the application using data examples based on real trials. A comprehensive simulation study shows that the proposed approach can maintain the true positive and false positive rates at desired levels. Possibility of added efficiency with be discussed. Perspectives will be also given on the future methodology development for basket designs.
|
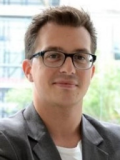
Olivier Collignon
|
Dr Olivier Collignon is Associate Director at GSK in the United Kingdom where he leads a team of statisticians who contribute to the development of clinical trials for immune-inflammatory diseases. He holds a PhD in Applied Mathematics and is especially interested in basket, umbrella and platform trials, use of historical controls and clinical prediction models. He previously worked as a biostatistician in France and Luxembourg for more than 15 years. During his mission at the Luxembourg Institute of Health he was seconded at the European Medicines Agency in London for 4 years, where he gained regulatory experience and participated to the scientific evaluation of the design and results of clinical trials to obtain marketing authorization of new drugs in Europe.
|
Estimands and Complex Innovative Designs.
Since the release of the ICH E9(R1) (International Council for Harmonisation of Technical Requirements for Pharmaceuticals for Human Use Addendum on Estimands and Sensitivity Analysis in Clinical Trials to the Guideline on Statistical Principles for Clinical Trials) document in 2019, the estimand framework has become a fundamental part of clinical trial protocols. In parallel, complex innovative designs have gained increased popularity in drug development, in particular in early development phases or in difficult experimental situations. While the estimand framework is relevant to any study in which a treatment effect is estimated, experience is lacking as regards its application to these designs. In a basket trial for example, should a different estimand be specified for each subpopulation of interest, defined, for example, by cancer site? Or can a single estimand focusing on the general population (defined, for example, by the positivity to a certain biomarker) be used? In the case of platform trials, should a different estimand be proposed for each drug investigated? In this work we discuss possible ways of implementing the estimand framework for different types of complex innovative designs. We consider trials that allow adding or selecting experimental treatment arms, modifying the control arm or the standard of care, and selecting or pooling populations. We also address the potentially data-driven, adaptive selection of estimands in an ongoing trial and disentangle certain statistical issues that pertain to estimation rather than to estimands, such as the borrowing of nonconcurrent information. We hope this discussion will facilitate the implementation of the estimand framework and its description in the study protocol when the objectives of the trial require complex innovative designs.
|
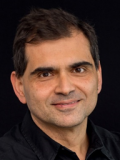
Pantelis Vlachos
|
Pantelis Vlachos is VP of Customer success in Cytel. He designs and implements clinical development programs and clinical trials, performs statistical analysis, and provides critical statistical input to support regulatory compliance. Pantelis has broad clinical research experience spanning early through late-stage clinical development and drug safety assessment in both large-scale pharmaceutical as well as academic settings. Prior to joining Cytel in 2013, Pantelis was a Principal Biostatistician at Merck Serono in Geneva, as well as a Professor of Statistics at Carnegie Mellon University for 12 years. He is a co-founder and former Managing Editor of Bayesian Analysis journal. He has also served on the editorial boards of various other journals, and online statistical data and software archives. Pantelis has contributed to the development of clinical development plans and provided statistical input to submission documents for regulatory authorities. His research interests lie in the area of adaptive designs, mainly from a Bayesian perspective, as well as hierarchical model testing and checking. He has applied his research in studies ranging from Phase I clinical pharmacology and dose escalation, Phase II dose finding, to Phase III studies in oncology, autoimmune disease, vaccines, and other. Pantelis contributes to software development and provides trainings for Cytel’s software products in Europe.
|
Simulation-based optimization of adaptive designs.
At Cytel we have historically been in the forefront of developing and implementing adaptive statistical methods for clinical trial design. Such methods along with the most recent developments from the industry have been materialized through the custom and commercial software we have released (Stat/Log-Xact, Compass, EAST, OkGO etc). In our most recent effort, we are utilizing the power of cloud computing and the custom statistical engines built over the years to create a tool that collects information from different parts of the clinical development team (clinical, operations, commercial etc) and with the statistician at the driver seat seeks and proposes designs that optimize a clinical study with respect to sample size, cost, duration and power. Furthermore, this tool can be used to communicate and update information to the trial team in real time, considering (possibly) changing target objectives. Case studies of actual adaptive trials will be given.
|